Advances in artificial intelligence (AI) are leading the charge in 21st century medical innovation and beginning to have real-world impact. AI is proving to be highly useful in the analysis of diagnostic imagery. Radiation oncology, organ allocation, robotic surgery and several other areas stand to benefit from its integration in the short to medium term. However, this raises significant issues of data usage and control, as many AI health technologies use large quantities of patient data.
Most health technologies are developed at public institutions, undergo a commercialization process and end up owned and controlled by private entities. As are the ones developed privately, of course. As AI self-improves, the way health technologies use the large quantities of patient data will change over time. Because AI itself is often opaque for purposes of oversight, a high level of engagement with the companies developing and maintaining the technology will be necessary. Some regulators, including the U.S. Food and Drug Administration, are certifying institutions that develop and maintain AI, rather than focusing on the ever-changing AI itself.
These public-private arrangements will necessitate placing more patient health information under the control of for-profit corporations. While this is not novel in itself, the structure of the public-private interface used in the implementation of health-care AI often will mean such corporations will have a greater than typical responsibility to obtain, utilize and protect patient health information. Thus the concerns about data usage and ongoing data security.
A significant portion of existing technology relating to machine learning and neural networks rests in the hands of large tech corporations. Google, Microsoft, IBM, Apple and others are all “preparing, in their own ways, bids on the future of health and on various aspects of the global health-care industry.”
We know that some recent public-private partnerships for implementing machine learning have resulted in poor privacy protection. For example, DeepMind, owned by Alphabet Inc. (i.e., Google), partnered with the Royal Free London NHS Foundation Trust in 2016 in the United Kingdom to use machine learning to assist in the management of acute kidney injury. Critics said that patients were not given control over the use of their information, nor were privacy impacts adequately discussed. A senior advisor with the Department of Health went so far as to say the patient info was obtained on an “inappropriate legal basis.” Further controversy arose after Google subsequently took direct control over DeepMind’s app, effectively transferring control over stored patient data from the U.K. to the United States. The ability to essentially “annex” mass quantities of private patient data to another jurisdiction is a new reality of big data and one at increased risk of occurring when implementing commercial health-care AI. The concentration of technological innovation and knowledge in big tech companies creates a power imbalance where public institutions can become more dependent and less equal and willing partners.
Beyond the possibility for general abuses of power, AI poses a novel challenge because the algorithms often require access to large quantities of patient data, may alter the scope of data used, and may use the data in different ways over time. The location and ownership of servers and computers that store and access patient health information are important in these scenarios. Regulation could require that patient data remain in the jurisdiction from which it is obtained, with few exceptions. This would also help decentralize control of the technology and distribute its economic benefits more widely.
Strong privacy protection is realizable when institutions are structurally encouraged to cooperate to ensure data protection by their very designs. The public-private partnership can be manageable for the purposes of protecting privacy but it introduces competing goals. Corporations may not be sufficiently encouraged to always maintain privacy protection if they can monetize the data or otherwise gain from them, and if the legal penalties are not high enough to deter this behaviour. Because of these and other concerns, there have been calls for greater systemic oversight of big data health research and technology.
Security breaches also are a major issue and are becoming more frequent in Canada. Storing huge quantities of patient health data at private institutions, whether scrubbed of personal identities or not, comes with risk. One risk is of privacy breaches caused by highly sophisticated AI that are making it harder to protect health information. A number of recent studies have highlighted emerging algorithmic methods for identifying individuals in health data repositories managed by public or private institutions, even when the data is deidentified. In other words, new AI can take anonymous data and combine it with other information to figure out which patient it applies to, and then use this experience to make itself even better at doing it next time. One study found that an algorithm could be used to re-identify 85.6 per cent of adults and 69.8 per cent of children in a physical activity cohort study “despite data aggregation and removal of protected health information.” A study from 2018 concluded that data collected by ancestry companies could be used to identify approximately 60 per cent of Americans of European ancestry and that the percentage was likely to increase substantially. In 2019, researchers successfully used a “linkage attack framework” that can link anonymized online health data to real-world people. These and other examples have raised questions about the security of health information framed as confidential. It has been suggested that these techniques “effectively nullify scrubbing and compromise privacy.”
This reality raises questions of liability, insurability and other practical issues that differ from instances where state institutions directly control patient data. Considering the variable and complex nature of the legal risk private AI developers could take on when dealing with high quantities of patient data, carefully constructed contracts will need to be made delineating the rights and obligations of the parties involved and liability for the various potential negative outcomes.
Canadian governments need to make better plans for dealing with the privacy issues concomitant with the implementation of big data-driven health-care AI. Our national policymaking in this arena is incomplete and lags behind several other countries, including the U.S. and several nations in the European Union. The federal government has working groups engaged on health-care AI regulation but has not finalized its guidance in the area and the provinces are a varied patchwork. As is often the case, the law risks falling far behind the technology.
Now that we are dealing with technologies that can improve themselves much faster than a human could, we risk falling very behind, very quickly.
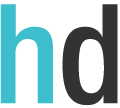
The comments section is closed.