As Artificial Intelligence technologies expand at an unprecedented rate, charting the unexplored frontiers of health-care AI has never been more urgent. In this third of a three-part series, we explore the nascent legal landscape of health-care AI, appraise the value of patient data and question the appropriate use of AI. Read the first article two articles here and here
“The world is run by male CEOs. Women are rarely doctors, lawyers or judges.”
After generating 5,000 unique, photorealistic images, this is what the world looks like to the Artificial Intelligence (AI) model Stable Diffusion. Although this finding contains a sad grain of truth – it was only in 2023 that all female CEOs from S&P 500 companies finally outnumbered CEOs with the first name John – it is clear that AI models can still fall victim to the biases of their human makers … and thus risk repeating our mistakes.
“Innovation will never be equitable,” sighs Ron Beleno, patient advocate and early health-tech AI adopter. “The digital divide will always be there when the tech comes.”
Medicine already has a bad rap for underrepresenting women and racial minorities in research and drug development, with potentially lethal consequences. Underrepresentation in data often leads to lower accuracy of results and conclusions. Feed those into AI, which (cluelessly) absorbs inherent biases alongside knowledge from the datasets the algorithms are trained on, and we have a fertile Petri dish for regurgitating the most exaggerated, distorted and shameful perceptions of our society.
AI see, AI do
Not only does data have to be vetted for biases and both internal and external validity, our behaviours as users provide another rich data repository. In the infancy of the AI age, all physicians become kindergarten teachers, unwittingly molding AI models through our very interactions with it.
“There are a lot of soft curriculum things that are not reliably captured from (electronic medical record) datasets – for example, teaching a medical student how to interpret ambiguous findings,” states Jaron Chong, radiologist and health-care AI expert on several national advisory boards. Chong notes that doctors’ clinical choices and institutional practices are learned – and copied – predominantly from AI observing the activities of users. As a result, AI can learn clinical cognitive biases as easily as it learns good hospital etiquette.
“[The AI] may not have a semantic understanding of the reasoning behind its conclusions,” Chong explains. As a result, extra caution is needed from users when interpreting its results, recognizing both human and machine fallibility for fallacies.
Similar to how bad human habits such as cognitive biases or incomplete admission orders may get passed onto AI, AI can also erroneously learn from the clinicians’ conclusions. Like an echo chamber, the action of the user implementing a specific AI-recommended data point creates another data point that reinforces the original decision made by the AI.
“The only antidote is higher quality experimentation, or higher quality data,” states Chong. “Humans have to be in the loop and be aware that this paradox can occur.”
Although AI’s voracious learning – regardless of good or bad, accurate or faulty – is the root of these aforementioned issues, it may also prove to be its panacea. AI’s greatest strength is the mutability and ever-changing nature of its algorithms – it easily can be course-corrected back to the safe and fallacy-free optimal.
But to address AI algorithms gone awry after exposure to the real world of messy patient encounters, post-market surveillance and regulations are needed.
Currently, Canada operates on what is a bare minimum – surveillance of adverse events, meaning that a product is legally obligated to reiterate its model only after an error or harm has been incurred. Chong says this is insufficient: “If you don’t have [ongoing auditing], then you find out [errors] on patients after market launch. We can’t afford that kind of mistake.”
Recommendations outlined in the jointly developed Canadian-U.S.-U.K. Guidelines for Good Machine Learning Practice emphasize that deployed AI models should have the “capability to be monitored in ‘real world’ use with a focus on maintained or improved safety and performance … with appropriate controls to manage risks of unintended bias or degradation of the model.”
Ongoing costs of surveillance and mandates for frequent reiteration, however, have the unintended consequence of chilling innovation. “If you raise the premarket regulation to an onerous level, you can have innovation avoiding your country. If there is no commercial profit, we deny Canadians access.” Chong states.
“If you raise the premarket regulation to an onerous level, you can have innovation avoiding your country.”
To minimize the burden, he recommends surveillance measures such as simulating the first month of use collected across a variety of algorithms. As for who is the most qualified to conduct surveillance: “The best folks to manage and monitor are the same people you rely on daily to ensure safety as a whole.”
In addition to established bodies like Health Canada, this may mean ongoing physician involvement in the AI development field, including timely clinical evidence-based updates, ongoing user feedback and course correction during clinical use. “As a clinical community, if we do not assert ourselves [in the process], then the status quo is [post-market surveillance] with adverse events,” says Chong.
See AI, do AI, teach AI
That, however, does not mean doctors will need to learn coding.
“The focus is on outputs,” emphasizes Chong, “We may not need to know the ins and outs of coding, but we do need to know how these algorithms might change the outcomes of our clinical practice.”
An analogy would be to treat AI as a drug. Just as physicians are expected to know the outcomes and side effects of prescribing a particular medication without needing to know the biochemical formulation, physicians can also apply specific AI models in a clinical context without knowing how to code.
Most health-care AI models are still assistive systems and require a human in the loop, though their mistakes may be quite alien in comparison to human error. As a radiologist, Chong provides an example from his field: “Windowing, contrast and noise can distort the performance of an AI model [in reading scans] but be trivial to a human. On the other hand, humans may have difficulty seeing a five-millimetre nodule.”
As a result, clinicians need to be aware of each AI model’s pitfalls. That may require academic research to characterize and validate models for reliability and sensitivity vs. specificity trends towards diagnosis. There may even be legal incentives for AI companies to skew toward sensitivity (resulting in more false positives) to reduce their liability over missed findings. Even in hypothetically “perfect” models without false positives or negatives, physicians need to be prepared for AI to unearth incidental findings that may or may not be clinically significant.
The addition of AI models to a clinician’s toolbox promises a sea change in daily clinical practice. It is not unimaginable that eventually, physicians may discuss at rounds the pros and cons of applying two different AI models the same way one currently decides between prescribing different diabetic oral medications. Whether this image is exhilarating or terrifying remains to be seen.
Not only will day-to-day clinical decisions be affected, but overall clinical standards of care may radically change.
There are plenty of historical precedents for technology upending what it means to be a good doctor. For instance, the advent of anesthesia allowed for more complicated, invasive and meticulous surgeries in which the skill of a surgeon is evaluated more on post-operative outcomes rather than the speed of one’s scalpel – previously necessary when operations were loud, bloody and limited by a patient’s pain tolerance.
Furthermore, Chong states that with the adoption of new technology, the balance between “specificity and sensitivity prioritization changes.” He notes that prior to abdominal imaging, surgeons would over-call for appendectomies because the alternative of ignoring a missed appendicitis was more dangerous. Once imaging helped rule out appendicitis, surgeons veered away from over-sensitivity, dropping the number of false-positive surgeries. Chong sums up the parallel succinctly: “The standards we practice at now are not the same expectations that will be in the future.”
Lifelong (machine) learning
As an early AI adopter when taking care of his father with dementia, patient advocate Beleno says new technology tends to become pervasive quickly – like the smartphone or Zoom video meetings – and that patients learn fast. “When people get really educated on AI, they will be questioning the health-care providers on what’s out there,” he says. “It’s a question of can health-care workers keep up?”
Much ink has been spilled on the self-learning capabilities of artificial intelligence. Not much has yet been said on physicians’ learning gap on AI in health care. As physicians become the link between health care and artificial intelligence, it is ever more important to bridge this gap. Physicians will need to understand the limitations of high-yield AI systems applied in a clinical setting, provide ongoing expert feedback to prevent post-market algorithmic drift, and recognize their role as canaries in the coal mine if health-care AI systems drift away from patient-centered priorities and incentives.
It is a daunting prospect. “You get nuance by being hands-on with [AI’s] use. You don’t gain that intuitive understanding until you interact with it directly,” Chong says.
“Don’t give up agency. It is our job and responsibility to navigate this roll out.”
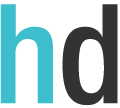
The comments section is closed.