Artificial intelligence is revolutionizing rehabilitation medicine, removing barriers inherent in traditional services and offering novel methods to some of the 2.4 billion people the World Health Organization estimates may benefit from rehabilitation.
Wearable sensors and risk prediction algorithms are among the applications for patients undergoing cardiac, stroke, neurological and physiotherapy rehabilitation.
Their advent offers appealing possibilities for the future of rehabilitation medicine that is projected to grow with the rising global burden of chronic disease and age-related disability.
One early example is an adaptive virtual assistant (AVA) developed by the KITE Research institute at Toronto’s University Health Network that functions as a virtual fitness trainer and was introduced while rehabilitation centres were closed during the pandemic. With video from a laptop or phone, the software tracks body joint movements and provides personalized exercise regimens and feedback. It provides real-time textual and progress bar feedback and comes with customizable background scenes to enhance accessibility.
“A lot of people live in remote regions, and there are lots of other financial barriers. There’s missed opportunities, and we wanted to create a solution that can be accessible even remotely,” says Shehroz Khan, Assistant Professor at the University of Toronto’s Institute of Biomedical Engineering and the lead of the virtual rehabilitation project at KITE.
Khan’s team visited patients’ homes to install the software and deliver training on the system. With biweekly check-ins, the team also conducts clinical assessments and provides patients with educational materials like printouts and leaflets.
“We made these systems very simple, so there is no additional hardware,” says Khan. “There’s only one button to start the data collection and patients can also stop the data collection.”
Meanwhile, in Illinois, Arun Jayaraman, Professor of Physical Medicine and Rehabilitation at Northwestern University’s Feinberg School of Medicine, and his team at Shirley Ryan AbilityLab have developed a stroke sensor toolkit to automate clinical outcome measures on inpatients. Within a maximum of five minutes, all clinical scores can be determined through a short sequence of activities termed “snapshot testing,” saving hours normally spent on conducting conventional tests.
At a hospital with 55,000 to 60,000 patients a year, “we do it very systemically because you want to do justice to the patients, which means you have to be super accurate. And to the clinicians, because you don’t want to misguide them,” says Jayaraman.
In home settings, remote monitoring of rehabilitation patients allows family members and care providers to set alerts for potential warning signs of an adverse health event. Stickable sensors are placed on the location pertinent to the data being collected, which are uploaded to a local receiver such as a phone or tablet and transferred to a secure cloud environment. The care team can set thresholds for changes in heart rate, blood pressure or EKG, for instance, that can trigger a text to the patient’s closest family member or a call to the local ambulance.
These warning systems can be particularly useful for older adults living on their own or in remote areas, where biomonitoring would otherwise be challenging.
These warning systems can be particularly useful for older adults living on their own or in remote areas.
“These patients, because of a lack of mobility, are often very fearful of going out and they’re kind of stuck at home. Social isolation and functional decline are kind of correlated together, and both of them, unfortunately, are not really part of primary care, unless you go to a physician and say, ‘Hey, I am socially isolated’,” says Khan.
Ideally, rehabilitation practitioners can adjust care specifically for each patient – a precision medicine approach in which the right treatment is given at the right time to the right patient.
This has always been a challenge, says Dan Lizotte, Associate Professor in Computer Science and Epidemiology and Biostatistics at Western University, because rehabilitation medicine requires a more sensitive decision-making paradigm that captures the complexities and uncertainties of long-term patient outcomes.
“If you think about how a lot of health research informs decision-making, a lot of it is single decisions considered in isolation and in a context where the options are relatively limited,” says Lizotte. “But that kind of isolated single decision point support is not adequate, because that’s not how physiotherapists are working to get their patients to the recovery that they want.”
At Parkwood Institute in London, Ont., Lizotte leads a study aiming to harness the methodological match between an area of AI known as reinforcement learning and the need to understand long-term impacts of decisions in rehabilitation medicine.
Rather than searching for a guideline that can be applied to all people at all times, the project aims to provide computerized decision support in clinical equipoise, moments when a clearly superior alternative does not exist.
“We could potentially break ties after the options have been narrowed down to say, ‘Based on the data we’ve collected and the trajectories of some patients, there might be evidence that one particular activity has been more successful in the past than another one.’”
While their applications in rehabilitation medicine are diverse, AI-driven tools have yet to be deployed on a widespread scale for reasons mostly related to economics, says Jayaraman. Cost savings are not readily visible in the short term.
Privacy concerns also exist.
“We have to be careful on how much information we provide or set some ground rules on whether insurance companies can misuse this information against patients who are vulnerable,” says Jayaraman.
Khan says he hopes the progress made in adapting AI tools for use in rehabilitation will demonstrate that policymakers should think about digital health seriously. While virtual rehabilitation sidesteps many of the barriers to traditional rehabilitation, AI-driven tools require a computer or mobile device and Internet access, which may create new barriers. Subsidizing WiFi routers and Internet services represents one way to ensure equitable access.
By restructuring the human-technology interface, AI-driven tools have the potential to change the scope and practice of rehabilitation medicine. However, the heterogeneity of patient needs and goals in rehabilitation means that quick, single decision-support models may lack generalizability.
“I think in AI there’s a tendency to try to work as fast as possible,” says Lizotte. “But it’s really looking at a whole field in health care and saying that if we’re going to think about evidence-based medicine in this setting, we need to take the long view because there are so many challenges that are surmountable but require consultation and long-term thinking.”
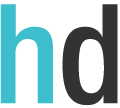
The comments section is closed.